Massive MIMO for Massive Access
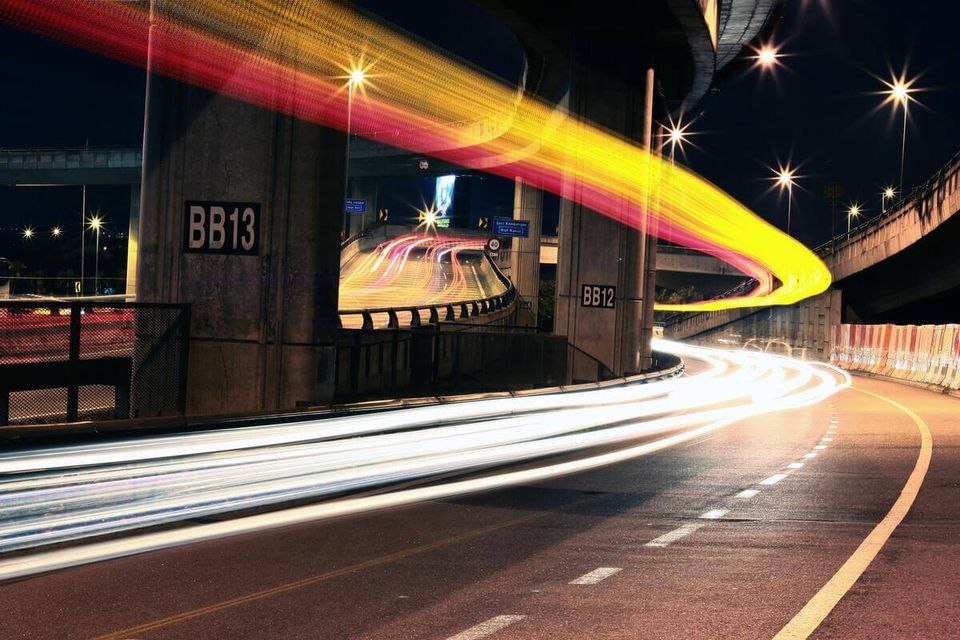
Introduction:
Massive multiple-input multiple-output (MIMO) is a promising technology for fifth-generation (5G) and beyond wireless communication systems that can provide high spectral efficiency, energy efficiency, and reliability. Massive MIMO systems use a large number of antennas at the base station (BS) and multiple user equipment (UE) to exploit the spatial domain for transmitting and receiving data. Massive MIMO can provide several benefits over traditional MIMO systems, including higher throughput, better signal quality, and improved interference management. However, massive MIMO faces several challenges and limitations, especially in scenarios with massive access, where a large number of UEs need to communicate simultaneously. In this article, we will discuss the technical aspects of massive MIMO for massive access, including the system model, channel estimation, signal processing, and resource allocation.
System Model:
The system model of massive MIMO for massive access can be described as follows. Let us consider a BS equipped with M antennas and N UEs, where each UE is equipped with a single antenna. The BS is assumed to be connected to a high-capacity backhaul network, such as fiber or millimeter-wave links, that can provide fast and reliable data transmission between the BS and the core network. The UEs are distributed over a given coverage area, and each UE wants to communicate with the BS simultaneously. The communication between the BS and the UEs is performed in a time-division duplex (TDD) mode, where the same frequency band is used for both uplink and downlink transmissions.
Channel Estimation:
One of the main challenges of massive MIMO for massive access is accurate channel estimation. Channel estimation refers to the process of estimating the channel coefficients between the BS and the UEs, which are required for optimal signal processing and resource allocation. In massive MIMO systems, the channel estimation becomes more challenging due to the increased number of antennas and UEs, which leads to a high-dimensional channel matrix that needs to be estimated accurately. Several techniques have been proposed for channel estimation in massive MIMO systems, including pilot-based methods, compressed sensing, and machine learning.
Pilot-based methods are the most common technique for channel estimation in massive MIMO systems. In pilot-based methods, the BS sends a known pilot sequence to the UEs, which use it to estimate the channel coefficients and send them back to the BS. The BS uses the estimated channel coefficients to perform optimal signal processing and resource allocation. However, pilot contamination is a major issue in pilot-based methods, where the pilot sequences from different UEs overlap and interfere with each other, leading to inaccurate channel estimation and reduced system performance.
Compressed sensing is another technique for channel estimation in massive MIMO systems that can exploit the sparsity of the channel matrix to reduce the pilot overhead and the interference. Compressed sensing requires fewer pilots than conventional pilot-based methods and can provide accurate channel estimation with lower complexity. However, compressed sensing requires sophisticated algorithms for sparse signal recovery and can be sensitive to the noise and the sparsity level of the channel matrix.
Machine learning is a promising technique for channel estimation in massive MIMO systems that can learn the channel characteristics from the data and adapt to the dynamic channel conditions. Machine learning can provide accurate channel estimation with low pilot overhead and can handle non-linear channel models and non-stationary channel conditions. However, machine learning requires large amounts of training data and complex algorithms, which can increase the system latency and overhead.
Signal Processing:
After channel estimation, the next step in massive MIMO for massive access is signal processing. Signal processing refers to the techniques used to detect, demodulate, and decode the data transmitted by the UEs and recover the original message. Signal processing in massive MIMO systems is performed at the BS using advanced algorithms that exploit the spatial diversity and the interference properties of the channel. The main signal processing techniques used in massive MIMO systems are maximum ratio combining (MRC), zero-forcing (ZF) precoding, and minimum mean square error (MMSE) precoding.
MRC is a simple signal processing technique that combines the signals received from different antennas using the channel coefficients as weights. MRC can provide significant gains in the signal-to-noise ratio (SNR) and the capacity of the system, especially in scenarios with rich scattering and high mobility. However, MRC is sensitive to the channel correlation and the interference, which can limit its performance in scenarios with massive access.
ZF precoding is another signal processing technique that can mitigate the interference and improve the system performance in scenarios with massive access. ZF precoding uses the inverse of the channel matrix to remove the interference and increase the SNR at the UEs. ZF precoding can provide significant gains in the capacity and the energy efficiency of the system, especially in scenarios with dense deployments and high interference. However, ZF precoding requires accurate channel estimation and can suffer from numerical instability and sensitivity to the noise and the channel correlation.
MMSE precoding is a more advanced signal processing technique that can provide optimal performance in massive MIMO systems, especially in scenarios with massive access. MMSE precoding uses the minimum mean square error criterion to design the precoding matrix that minimizes the mean square error between the transmitted and received signals. MMSE precoding can provide significant gains in the capacity and the robustness of the system, especially in scenarios with high interference and non-uniform channel conditions. However, MMSE precoding requires high computational complexity and high overhead for channel estimation and feedback.
Resource Allocation:
The final step in massive MIMO for massive access is resource allocation. Resource allocation refers to the process of assigning the available resources, such as time, frequency, and power, to the UEs to optimize the system performance and satisfy the quality-of-service (QoS) requirements. Resource allocation in massive MIMO systems is performed at the BS using advanced algorithms that take into account the channel conditions, the interference, and the QoS constraints.
The main resource allocation techniques used in massive MIMO systems are orthogonal frequency division multiple access (OFDMA), non-orthogonal multiple access (NOMA), and rate-splitting multiple access (RSMA).
OFDMA is a well-established resource allocation technique that divides the frequency band into orthogonal subcarriers and assigns each subcarrier to a single UE. OFDMA can provide simple and efficient resource allocation, especially in scenarios with low interference and uniform channel conditions. However, OFDMA can suffer from the inter-carrier interference (ICI) and the inter-symbol interference (ISI) in scenarios with non-uniform channel conditions and high mobility.
NOMA is a more advanced resource allocation technique that can provide higher spectral efficiency and capacity in scenarios with massive access. NOMA uses power-domain multiplexing to allow multiple UEs to share the same frequency and time resources by using different power levels. NOMA can provide significant gains in the capacity and the energy efficiency of the system, especially in scenarios with dense deployments and non-uniform channel conditions. However, NOMA requires accurate channel estimation and high overhead for power allocation and feedback.
RSMA is a recently proposed resource allocation technique that can provide a good balance between simplicity and efficiency in massive MIMO systems with massive access. RSMA uses the rate-splitting concept to divide the data stream of each UE into two parts, one part that is decoded using MRC or ZF precoding, and another part that is decoded using RSMA. The RSMA part is further split into two sub-streams, one sub-stream that is decoded using ZF precoding, and another sub-stream that is decoded using successive interference cancellation (SIC). RSMA can provide significant gains in the capacity and the robustness of the system, especially in scenarios with high interference and non-uniform channel conditions. Moreover, RSMA can reduce the complexity and overhead of the system compared to NOMA and MMSE precoding.
Conclusion:
Massive MIMO for massive access is a promising technology that can provide significant gains in the capacity, the energy efficiency, and the user experience of 5G and beyond networks. Massive MIMO can exploit the spatial diversity, the beamforming, and the interference management to increase the number of connected devices and the data rates, while maintaining the quality-of-service requirements.
Massive MIMO for massive access involves several technical challenges, such as antenna design, channel estimation, signal processing, and resource allocation. These challenges require advanced techniques and algorithms that take into account the complex and dynamic nature of the wireless environment and the diverse needs of the users.
Antenna design is critical to the performance of massive MIMO systems and involves optimizing the antenna array size, shape, and orientation to maximize the spatial diversity and the beamforming. Channel estimation is another critical aspect of massive MIMO systems and involves accurate and efficient estimation of the channel matrix using pilot signals and advanced algorithms.
Signal processing in massive MIMO systems involves advanced techniques such as MRC, ZF precoding, and MMSE precoding, which can mitigate the interference and increase the SNR and the capacity of the system. Resource allocation in massive MIMO systems involves advanced techniques such as OFDMA, NOMA, and RSMA, which can optimize the allocation of the available resources to the UEs to satisfy the QoS requirements and increase the capacity and the energy efficiency of the system.
Overall, massive MIMO for massive access is a promising technology that can enable the vision of 5G and beyond networks to connect everything and everyone, everywhere and all the time. However, massive MIMO for massive access requires further research and development to overcome the technical challenges and realize its full potential in real-world scenarios.