How does edge intelligence contribute to the efficiency of 6G systems?
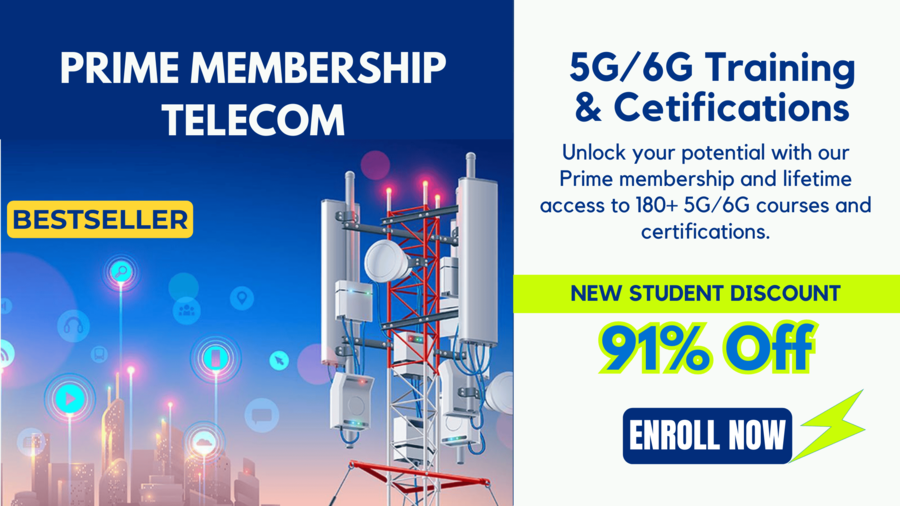
Edge intelligence refers to the deployment of computational and analytical capabilities closer to the edge of a network, near the data source or end-user device, rather than solely relying on centralized cloud-based systems. This approach significantly reduces latency, enhances real-time processing, and improves the overall efficiency of network systems. 6G systems, edge intelligence plays a crucial role in several ways:
- Reduced Latency: By processing data closer to the source, edge intelligence minimizes the time it takes for information to travel back and forth between devices and centralized servers. This reduced latency is critical for time-sensitive applications like autonomous vehicles, augmented reality (AR), virtual reality (VR), remote healthcare, and industrial automation where split-second decision-making is essential.
- Bandwidth Optimization: Edge intelligence enables data preprocessing and filtering at the edge devices before transmitting information to the central cloud. This helps in optimizing bandwidth usage by transmitting only essential data, reducing congestion, and optimizing network resources.
- Enhanced Privacy and Security: Keeping data processing closer to the edge ensures that sensitive information can be processed locally, reducing the need to transmit it across networks. This minimizes the risk of data breaches and enhances privacy by keeping sensitive data within a more controlled environment.
- Improved Reliability and Resilience: Edge intelligence allows for decentralized processing, which means even if there's a network outage or disruption, certain critical functions can still operate independently at the edge. This enhances the system's overall reliability and resilience.
- Support for Massive IoT (Internet of Things) Deployment: 6G networks are expected to handle a massive number of IoT devices. Edge intelligence facilitates efficient management, processing, and coordination of the vast amount of data generated by these devices by distributing processing closer to the data source, reducing the burden on the core network.
- AI and Machine Learning at the Edge: The integration of AI and machine learning models at the edge enables real-time decision-making and analysis without relying heavily on cloud-based processing. This allows for rapid insights and actions based on locally processed data, improving system efficiency and responsiveness.
- Customization and Personalization: Edge intelligence enables personalized services and content delivery by analyzing user behavior and preferences at the edge. This customization can be achieved in real-time, providing a more tailored experience for users.