Explain the role of machine learning and artificial intelligence in 5G network planning.
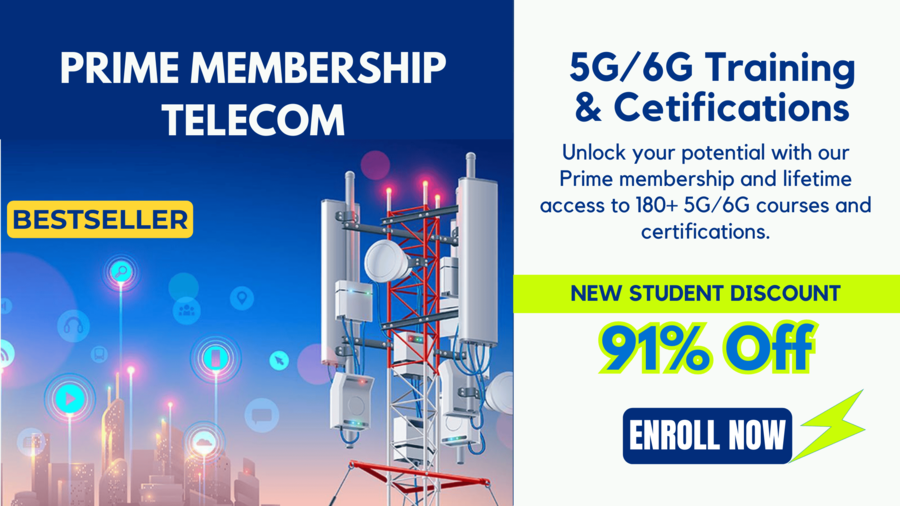
Machine learning (ML) and artificial intelligence (AI) play crucial roles in the planning, optimization, and management of 5G networks. 5G, the fifth generation of wireless technology, introduces several advancements such as increased data rates, lower latency, massive device connectivity, and network slicing. ML and AI technologies are leveraged to address the complexities and challenges associated with the deployment and operation of these advanced networks. Here's a detailed technical explanation of their roles in 5G network planning:
- Radio Frequency (RF) Planning:
- Problem Statement: Efficient allocation of radio frequencies is critical in 5G to ensure optimal coverage, capacity, and performance.
- Role of ML/AI: ML algorithms can analyze vast amounts of data related to terrain, user behavior, and network performance to predict optimal frequency allocation. AI can dynamically adjust frequency assignments based on real-time changes in the environment, user demand, and interference patterns.
- Network Slicing:
- Problem Statement: 5G networks support network slicing, where virtual networks are created to meet specific service requirements. Planning and managing these slices are complex tasks.
- Role of ML/AI: AI helps in automated and dynamic allocation of resources to different slices based on the changing demands. ML models can predict the resource requirements for various slices, enabling efficient allocation and optimization.
- Massive MIMO (Multiple Input Multiple Output):
- Problem Statement: 5G utilizes massive MIMO technology to increase spectral efficiency and capacity, but optimizing the deployment of a large number of antennas is challenging.
- Role of ML/AI: ML algorithms can analyze historical data, predict user mobility patterns, and optimize the beamforming of massive MIMO systems. AI can dynamically adjust beamforming parameters to adapt to changing environmental conditions and user distribution.
- Self-Healing Networks:
- Problem Statement: 5G networks need to be resilient and capable of self-healing to address issues such as equipment failures or sudden increases in traffic.
- Role of ML/AI: AI-based fault prediction models can anticipate potential issues, while ML algorithms can analyze network performance data to detect anomalies. AI-driven automation can then trigger self-healing mechanisms, such as rerouting traffic or adjusting parameters, to maintain network integrity.
- Energy Efficiency:
- Problem Statement: With the increase in network complexity, energy consumption becomes a significant concern for 5G networks.
- Role of ML/AI: ML models can analyze historical data and predict network traffic patterns, allowing AI algorithms to optimize the energy consumption of network elements. This involves dynamically adjusting the power levels of base stations and other network components based on real-time demand.
- Dynamic Resource Management:
- Problem Statement: The dynamic nature of 5G requires efficient allocation and management of resources like bandwidth and processing power.
- Role of ML/AI: ML models can learn from historical usage patterns and predict resource demands. AI algorithms can then dynamically allocate and optimize resources to meet the requirements of different services and applications, ensuring efficient use of network resources.
Machine learning and artificial intelligence enable 5G network planners and operators to automate complex tasks, optimize resource allocation, enhance network performance, and respond dynamically to changing conditions, ultimately providing a more efficient and reliable 5G network.