Bringing AI research to wireless communication and sensing
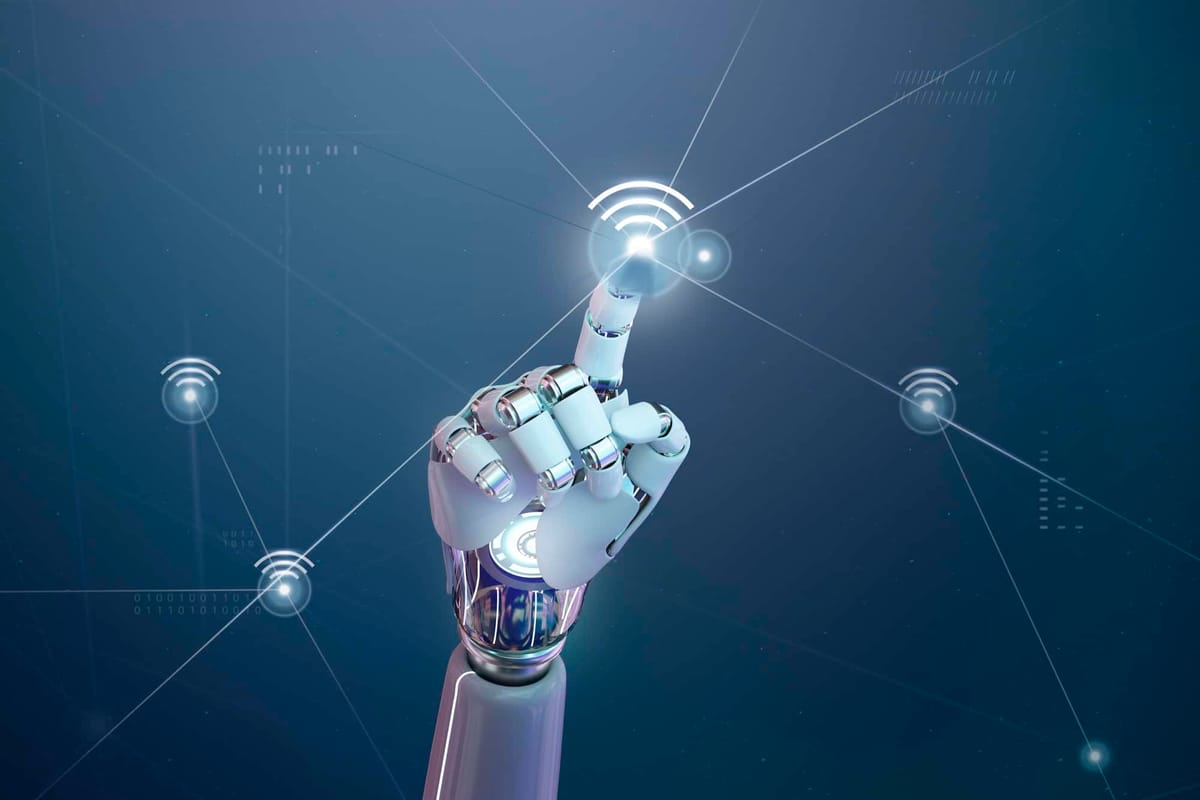
Wireless communication and sensing technologies have rapidly evolved in recent years, enabling a wide range of applications across different sectors including healthcare, transportation, and manufacturing. The integration of Artificial Intelligence (AI) techniques into these technologies has the potential to further enhance their capabilities and provide more accurate, efficient, and reliable solutions.
In this article, we will discuss the technical aspects of bringing AI research to wireless communication and sensing technologies. We will explore some of the key challenges, opportunities, and applications of this integration.
Challenges
There are several technical challenges that need to be addressed in order to successfully integrate AI into wireless communication and sensing technologies. These include:
- Data Acquisition and Processing: Wireless communication and sensing technologies generate large amounts of data that need to be processed and analyzed in real-time. AI techniques can help to process this data and extract meaningful insights. However, the quality and accuracy of the data can be affected by several factors such as noise, interference, and signal attenuation. Therefore, it is crucial to develop robust data acquisition and processing techniques that can handle these challenges.
- Power Consumption: Wireless communication and sensing devices are typically battery-powered, and their power consumption can have a significant impact on their performance and lifespan. AI algorithms require significant computational resources, which can increase the power consumption of these devices. Therefore, it is important to develop energy-efficient AI algorithms that can operate within the power constraints of these devices.
- Latency: Latency is the time delay between the generation and reception of a signal, and it can be a critical factor in many wireless communication and sensing applications. AI algorithms can introduce additional processing time, which can increase the latency of these systems. Therefore, it is important to develop real-time AI algorithms that can operate within the latency constraints of these applications.
Opportunities
Despite the challenges, the integration of AI into wireless communication and sensing technologies presents several opportunities for improving the performance and capabilities of these systems. Some of the key opportunities include:
- Enhanced Sensing and Monitoring: AI techniques can help to extract more accurate and meaningful information from wireless sensor data, enabling better sensing and monitoring of physical systems. For example, in healthcare, AI can be used to analyze medical sensor data and provide early detection of diseases or health conditions.
- Improved Communication Efficiency: AI can be used to optimize wireless communication protocols, enabling faster and more reliable communication between devices. This can improve the efficiency and performance of wireless networks, enabling the development of new applications such as smart cities and autonomous vehicles.
- Predictive Maintenance: AI techniques can be used to analyze sensor data from machines and predict when maintenance is needed. This can help to reduce downtime and maintenance costs, improving the reliability and efficiency of manufacturing and industrial processes.
Applications
The integration of AI into wireless communication and sensing technologies has a wide range of applications across different sectors. Some of the key applications include:
- Healthcare: Wireless sensors can be used to monitor vital signs and other health parameters of patients. AI techniques can be used to analyze this data and provide early detection of diseases or health conditions, enabling better healthcare outcomes.
- Smart Cities: Wireless communication and sensing technologies can be used to monitor traffic, air quality, and other environmental factors in urban areas. AI techniques can be used to analyze this data and provide insights that can be used to improve the efficiency and sustainability of cities.
- Transportation: Wireless communication and sensing technologies can be used to enable vehicle-to-vehicle (V2V) and vehicle-to-infrastructure (V2I) communication, enabling safer and more efficient transportation systems. AI techniques can be used to optimize traffic flow and reduce congestion, improving the performance and reliability of transportation networks.
- Industrial Automation: Wireless sensors can be used to monitor machines and equipment in industrial settings. AI techniques can be used to analyze this data and predict when maintenance is needed, reducing downtime and maintenance costs. Additionally, AI can be used to optimize manufacturing processes and improve overall efficiency.
- Agriculture: Wireless sensors can be used to monitor soil moisture levels, crop growth, and other environmental factors in agriculture. AI techniques can be used to analyze this data and provide insights that can be used to optimize crop yields and reduce water usage.
Technical Approaches
There are several technical approaches that can be used to integrate AI into wireless communication and sensing technologies. Some of the key approaches include:
- Machine Learning: Machine learning techniques can be used to analyze wireless sensor data and extract meaningful insights. Supervised learning algorithms can be used to train models that can classify sensor data into different categories, while unsupervised learning algorithms can be used to identify patterns and anomalies in the data.
- Deep Learning: Deep learning techniques, such as neural networks, can be used to analyze wireless sensor data and extract more complex and abstract features. These techniques can be particularly effective in applications where the data is highly complex or noisy.
- Reinforcement Learning: Reinforcement learning techniques can be used to optimize wireless communication protocols by training agents to make decisions that maximize some performance metric, such as throughput or latency. These techniques can be used to develop more efficient and reliable wireless networks.
- Edge Computing: Edge computing involves processing data at the edge of the network, closer to the sensors and devices generating the data. This can help to reduce the latency and bandwidth requirements of AI algorithms, enabling real-time processing of wireless sensor data.
- Cognitive Radio: Cognitive radio is a wireless communication technology that uses AI techniques to optimize the use of available radio spectrum. These techniques can be used to dynamically allocate spectrum to different devices and applications, improving the efficiency and performance of wireless networks.
Conclusion
The integration of AI into wireless communication and sensing technologies has the potential to revolutionize many different industries and applications. However, there are several technical challenges that need to be addressed in order to fully realize these benefits. By developing robust data acquisition and processing techniques, energy-efficient AI algorithms, and real-time processing capabilities, we can enable more accurate, efficient, and reliable wireless communication and sensing systems. With continued research and development, we can unlock the full potential of AI in wireless communication and sensing technologies and drive innovation across a wide range of industries and applications.